Lifecycle vs. Lineage Explained
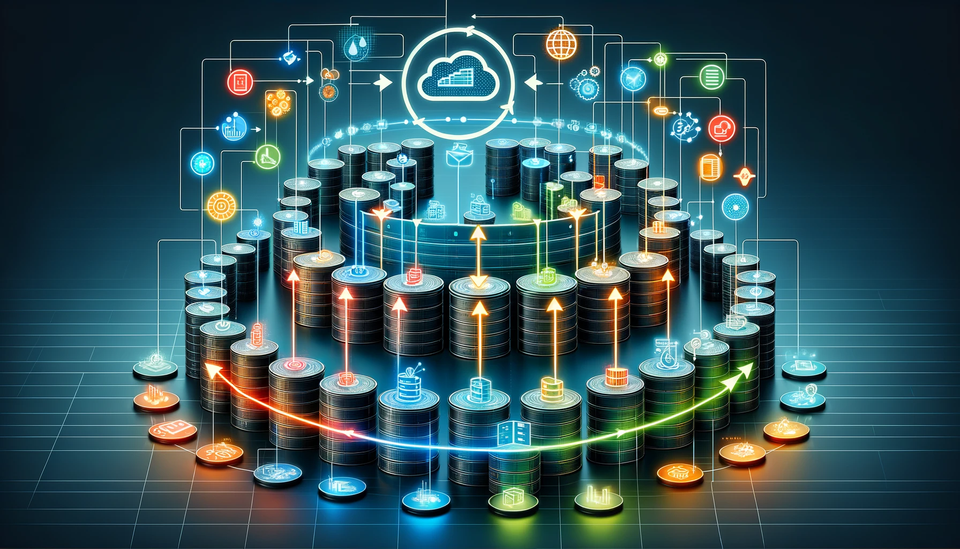
Data is the backbone of decision-making and understanding the nuances of data management is paramount in leveraging it effectively. Two critical concepts, often misunderstood or used interchangeably, stand at the core of robust data governance: the data lifecycle and data lineage. This article aims to define these terms, highlighting their distinct roles and the areas where they intersect. By exploring the differences and overlaps between the data lifecycle—the journey of data from creation to disposal—and data lineage—the history of data as it moves and transforms across systems—we provide clarity on their unique contributions to data governance. This understanding is not merely academic; it is a practical necessity for ensuring data integrity, compliance, and operational efficiency. Through this exploration, our purpose is to equip professionals with the knowledge to harness both concepts for improved data management, thereby enhancing the strategic value of their organization's data assets.
The Basics of Data Lifecycle
The data lifecycle encompasses the complete journey of data from its inception to its eventual retirement. Understanding this lifecycle is crucial for organizations aiming to maximize data's value while ensuring its integrity and compliance with regulations. The lifecycle can be broken down into several key stages:
- Creation or Collection: This initial stage involves generating or acquiring data from various sources, including transactions, user interactions, and external data feeds.
- Storage: Once created or collected, data needs to be securely stored in a way that balances accessibility with protection. This involves choosing the right storage solutions and formats that facilitate easy retrieval and use.
- Usage: Data is then utilized for various purposes, such as analysis, decision-making, and operational processes. Effective data lifecycle management ensures that data is accurate, up-to-date, and available to authorized users when needed.
- Sharing: Data may be shared internally within an organization or externally with partners and stakeholders. This stage requires careful management to maintain data security and privacy.
- Archiving: To keep data accessible for future reference or compliance purposes, it is archived. Archiving involves moving data to less expensive storage solutions while keeping it retrievable.
- Destruction: Finally, when data is no longer needed or is required to be disposed of due to regulatory requirements, it undergoes secure destruction to protect sensitive information from unauthorized access.
Effectively managing the data lifecycle is fundamental to data governance, as it ensures that data is handled efficiently and responsibly throughout its existence. Organizations that master data lifecycle management can improve their operational efficiency, enhance data quality, and achieve better compliance with data protection regulations, ultimately leveraging data as a strategic asset.
The Basics of Data Lineage
Data lineage refers to the detailed history of data, tracing its origins and journey through various transformations and movements across the data ecosystem. It is a critical component of data management, offering transparency and accountability for data quality, governance, and compliance. Understanding data lineage allows organizations to track the source of their data, understand how it has been altered, and identify who has interacted with it, providing a comprehensive view of this evolution.
The key aspects of data lineage include:
- Origin: Identifying where the data was initially created or sourced from, including external data providers, internal databases, or data entry points.
- Transformation: Documenting any changes made to the data, such as aggregations, filtering, or cleansing operations. This includes not only the transformations themselves but also the rationale behind them and the tools or processes used.
- Movement: Tracking the flow of data between systems, applications, and storage locations. This helps in understanding how data is shared across an organization and can highlight potential bottlenecks or points of data leakage.
- Usage: Recording how and where the data is used within the organization, including in reports, analytics, decision-making processes, and operational activities.
- Impact Analysis: Facilitating the assessment of how changes to one piece of data can affect downstream processes or datasets. This is crucial for risk management and ensuring data integrity.
Data lineage provides a foundation for trust in data by making its history transparent and accessible. It enables organizations to quickly diagnose and rectify issues, comply with regulations by providing audit trails, and make informed decisions based on reliable data. Moreover, effective lineage tracking supports data governance initiatives by ensuring that data usage aligns with organizational policies and standards.
Comparing and Contrasting
Data lifecycle and data lineage address different aspects of data management, focusing on the temporal management and the historical evolution of data, respectively. However, comparing and contrasting them reveals both distinct characteristics and areas of overlap, highlighting their unique and complementary roles.
- Data Lifecycle: This concept applies to an individual instance of data and encompasses its entire lifespan, from creation to deletion. It focuses on how data is physically stored, accessed, used, and eventually disposed of or archived. The lifecycle perspective is concerned with the stages through which data passes and the policies and practices for managing data at each stage to ensure its integrity, availability, and confidentiality.
- Data Lineage: Contrarily, data lineage pertains to the evolution of data as it moves through various processes and transformations, potentially across different storage locations and for various purposes. Lineage tracks the data’s journey, documenting how it has been altered, combined, split, or aggregated to serve different analytical or operational needs. This concept provides insight into the data’s origins and transformations, supporting traceability, compliance, risk management, and the understanding of how end products or analytical results are derived.
In summary, the data lifecycle is about the physical and temporal aspects of data management within its environment, emphasizing the "what" and "where" aspects of data at a specific point in time. Data lineage, on the other hand, focuses on the "how" and "why" of data’s transformations and movements across the data ecosystem, providing a historical account of its journey to its current state for different products and end results. Together, they provide a comprehensive view of data’s management and evolution, crucial for effective data governance and utilization.
Practical Applications and Best Practices
Integrating both data lifecycle and data lineage into data management practices offers tangible benefits for organizations, enhancing compliance, data quality, and decision-making. Here are practical applications and best practices for effectively managing these aspects:
Practical Applications
1. Regulatory Compliance and Auditing: Data lineage tools can automatically generate audit trails, showing the data’s origin, transformations, and who accessed it, essential for compliance with regulations like GDPR and HIPAA. Lifecycle management ensures data is retained and disposed of according to legal requirements.
2. Data Quality Improvement: By understanding the entire journey of data through lineage tracking, organizations can pinpoint and correct errors at their source. Lifecycle management practices, such as regular reviews of data for accuracy and relevancy, further bolster data quality.
3. Impact Analysis: Before implementing changes in data management processes or systems, data lineage allows organizations to conduct thorough impact analyses, predicting how changes will affect downstream processes and data quality.
Best Practices
1. Define Clear Policies and Procedures: Establish comprehensive policies for data lifecycle management, including data creation, storage, use, sharing, archiving, and destruction. Similarly, implement standards for documenting data lineage, ensuring consistency and reliability in data handling.
2. Leverage Technology Solutions: Utilize specialized software tools that support data lineage tracking and lifecycle management. These tools can automate many aspects of the processes, providing real-time visibility and control over data.
3. Foster a Culture of Data Governance: Encourage collaboration among data stakeholders (IT, legal, business units) to ensure a unified approach to managing data lifecycle and lineage. Promote awareness and training on the importance of data governance practices.
4. Regular Audits and Reviews: Conduct regular audits of data management practices and reviews of data lineage and lifecycle processes to identify areas for improvement. This proactive approach helps maintain compliance and data integrity.
5. Integration with Data Governance Framework: Ensure that data lifecycle management and lineage tracking are integral parts of the organization’s overall data governance framework. This alignment ensures that data policies support business objectives and compliance requirements.
By adopting these practices, organizations can create a robust framework for data management that not only complies with regulatory demands but also supports strategic decision-making and operational efficiency.
Leveraging Lifecycle and Lineage for Strategic Advantage
Mastering the management of data lifecycle and lineage provides organizations with a strategic edge, transforming data from a mere operational necessity into a pivotal asset for innovation, decision-making, and competitive advantage. Here's how businesses can leverage these concepts for strategic gain:
Enhancing Decision-Making and Analytics
- Data Integrity and Trust: By ensuring data is accurately tracked through its lifecycle and lineage, organizations can rely on the integrity and accuracy of their data. This trustworthiness is critical for analytics and decision-making, enabling businesses to make informed decisions based on reliable data.
- Granular Insight: Data lineage offers granular insights into data origins and transformations, aiding in more nuanced analysis and interpretation. Understanding the full context of data enhances analytical outcomes, leading to more effective strategic planning.
Improving Efficiency and Reducing Costs
- Streamlined Data Management: Effective lifecycle management helps in identifying redundant or obsolete data, enabling its removal or archiving. This not only reduces storage costs but also improves system performance by eliminating unnecessary data clutter.
- Automated Compliance: Automating aspects of data lifecycle and lineage tracking helps in maintaining compliance with less manual effort, significantly reducing the costs and risks associated with regulatory requirements.
Fostering Innovation
- Data as a Service (DaaS): With a clear understanding of data lineage, organizations can package and offer their data internally or externally as a service. This approach can unlock new business models and revenue streams by monetizing valuable data assets.
- Enhanced Collaboration: Knowing the lifecycle and lineage of data facilitates better collaboration between teams. When stakeholders understand where data comes from and how it’s processed, they can more effectively share insights and innovations.
Risk Management and Security
- Proactive Risk Identification: Tracking data lineage helps in identifying potential risks in data processes and sources. By understanding how data flows and transforms, organizations can anticipate and mitigate risks before they impact business operations.
- Data Breach Prevention: Lifecycle management practices ensure that sensitive data is securely handled, stored, and destroyed, reducing the risk of data breaches and ensuring data privacy.
Conclusion
Understanding and implementing data lifecycle and data lineage practices are essential for effective data management. These concepts serve distinct yet complementary roles: lifecycle management ensures data is handled efficiently from creation to deletion, while lineage provides crucial visibility into data’s history and transformations. Together, they enable organizations to maintain data quality, comply with regulations, and make informed decisions. As data becomes increasingly central to business operations, the ability to manage its flow and evolution strategically becomes a critical asset. Embracing these practices equips businesses to navigate the complexities of modern data environments, mitigate risks, and capitalize on data-driven opportunities. Looking ahead, the focus on lifecycle and lineage will be key for organizations aiming to leverage data responsibly and competitively.
Member discussion