Navigating the Path to Data Mastery: Understanding the Data Maturity Model
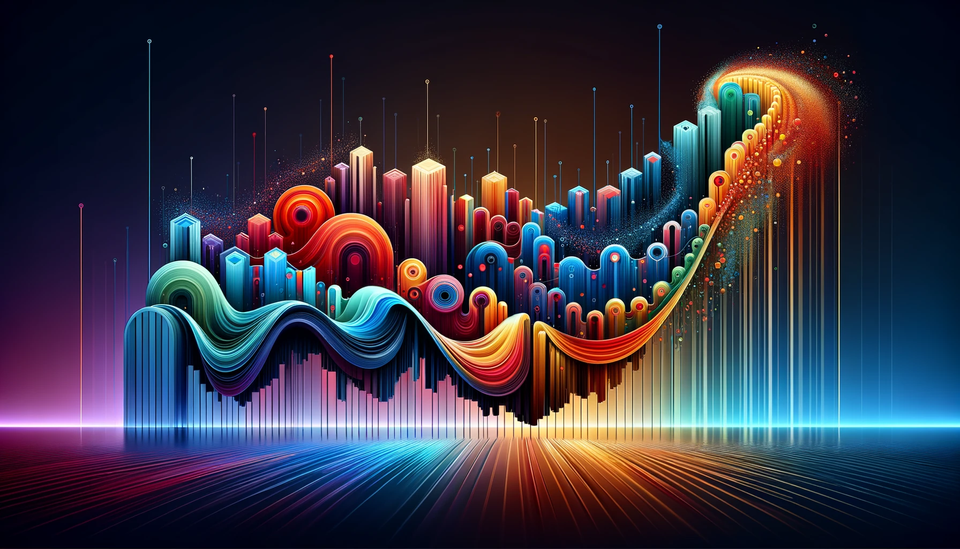
Introduction
In today’s data-driven business landscape, the ability to effectively manage and utilize data is not just an advantage; it’s a necessity. From multinational corporations to small businesses, the journey towards data maturity is crucial for making informed decisions, driving innovation, and maintaining a competitive edge. This journey, however, is layered and complex, involving various stages of growth and development in how data is governed, managed, and leveraged.
In this article, we will delve into the intricacies of the Data Maturity Model – a framework that outlines the evolutionary path organizations follow in their quest to achieve data excellence. We will break down each component of the model, from Data Governance and Stewardship to Analytics and Business Intelligence, providing insights into the distinct stages of maturity: Initial, Developing, Defined, Managed, and Optimized.
Join us as we unravel the layers of the Data Maturity Model, offering a roadmap for organizations to navigate the complex but rewarding journey towards data mastery.
Data Maturity Stages
The various levels of a data management maturity model can be categorized into distinct stages. Each stage represents a level of maturity in handling and utilizing data within an organization. Here's a naming convention that could be used to describe these stages:
- Initial Stage (or Nascent Stage)
- Characteristics: This is the starting point where data management practices are typically ad-hoc, unstructured, and reactive. There's a lack of formal policies or understanding of the value of data.
- Focus: Recognizing the importance of data and beginning to establish basic data handling procedures.
- Developing Stage (or Emergent Stage)
- Characteristics: The organization starts to recognize the importance of data management. Initial policies and roles are being established, but they are not yet fully integrated or consistent across the organization.
- Focus: Building foundational data management practices and starting to address data quality and governance issues.
- Defined Stage (or Structured Stage)
- Characteristics: Formal data management policies and procedures are in place. Roles and responsibilities related to data are clearly defined and understood across the organization.
- Focus: Ensuring consistent application of data policies and integrating data management into business processes.
- Managed Stage (or Advanced Stage)
- Characteristics: Data management practices are integrated into everyday business operations. There's active monitoring and enforcement of data policies, and data-driven decision-making becomes more prevalent.
- Focus: Refining data management strategies, aligning them closely with business objectives, and leveraging data for operational efficiency and strategic advantage.
- Optimized Stage (or Leading-Edge Stage)
- Characteristics: The organization exhibits advanced data management capabilities, with proactive and sophisticated strategies in place. Data management is continuously improved and is an integral part of the business strategy.
- Focus: Staying ahead of emerging trends, using cutting-edge technologies (like AI and ML) in data management, and continuously innovating and improving data practices.
Each stage represents a progression in the organization’s maturity in managing data, with the ultimate goal being to reach the Optimized Stage, where data management is a key driver of business success and innovation.
Data Maturity Model Dimensions
In a maturity model, the overarching categories or domains that encompass these stages are typically referred to as "dimensions" or "capabilities." Each dimension represents a specific area of focus or a key aspect of the broader subject the model addresses. In the context of a data management maturity model, these dimensions might include:
- Governance and Stewardship: This dimension focuses on how data is governed and managed within the organization, including policies, roles, and responsibilities related to data.
- Metadata and Reference Data Management: This area focuses on organizing and maintaining metadata (data about data) and reference data (standardized data sets) to ensure uniform understanding and usage across the organization, critical for effective data governance and analysis.
- Quality Management: This aspect deals with the processes and practices in place to ensure the accuracy, consistency, and reliability of data.
- Integration and Interoperability: This dimension assesses the organization's ability to integrate data from various sources and ensure that different systems can effectively communicate and exchange data.
- Storage and Architecture: This area looks at how data is stored and managed, including the infrastructure and technologies used for data storage and management.
- Security and Compliance: This dimension evaluates how data security and compliance with relevant laws and regulations are managed.
- Culture and Literacy: This aspect considers the organizational culture regarding data, including how data literacy is promoted among employees and how data-driven the organization's decision-making processes are.
- Analytics and Business Intelligence: This dimension assesses the use of data for analytics and business intelligence, including the tools and processes in place for data analysis.
Within each dimension, the stages (Initial, Developing, Defined, Managed, and Optimized) represent the levels of maturity an organization can achieve. These stages provide a roadmap for progression, helping organizations identify their current level of maturity in each dimension and what steps they need to take to advance to the next level.
Furthermore, these dimensions can sometimes be supported by "sub-dimensions" or "elements," which are specific areas of focus within each main dimension. For example, in the "Governance and Stewardship" dimension, sub-dimensions could include policy development, role definition, and data stewardship practices.
In summary, in a data management maturity model:
- Stages are the levels of maturity (Initial, Developing, Defined, Managed, Optimized) within each dimension.
- Dimensions/Capabilities are the broad areas of focus, such as Governance, Quality Management, etc.
- Sub-Dimensions/Elements are specific focus areas within each dimension, providing more detailed guidance on what each stage entails.
Data Management Roadmap
Creating a data management roadmap that demonstrates progress from low to high maturity involves organizing it around key topics that are critical to the evolution and sophistication of data management practices. These topics, represented by the dimensions, in combination with the maturity stages, should reflect the progression in capabilities, governance, and integration of data management within the organization. Here's a structured approach:
Data Governance and Stewardship
Data Governance and Stewardship form the foundational framework that dictates how data is managed, protected, and utilized within an organization. This crucial dimension encompasses the establishment of policies, procedures, standards, and roles aimed at ensuring data accuracy, accessibility, consistency, and security. Data Governance provides the overarching structure and guidelines under which data operations are conducted, ensuring compliance with legal and regulatory requirements, while Data Stewardship involves the practical management and oversight of data assets by designated individuals or teams. Together, they ensure that data is treated as a valuable and strategic asset, facilitating trust among stakeholders, enhancing decision-making processes, and supporting the organization's objectives. Effective Data Governance and Stewardship are essential for maintaining data quality, fostering a culture of data responsibility, and enabling organizations to leverage their data for competitive advantage.
- Initial Stage: At this stage, organizations lack a formal approach to data governance. Data handling is often ad-hoc, reactive, and decentralized, leading to inconsistencies and potential data integrity issues. There's little awareness of the risks associated with poor data management, and data stewardship roles are either non-existent or not clearly defined.
- Developing Stage: The organization begins to recognize the need for structured data governance. Basic policies are drafted to provide some level of consistency in data handling. Initial roles like data stewards are identified, albeit with limited authority and scope. These stewards start to take responsibility for data quality and accessibility within specific departments, but a company-wide approach is still lacking.
- Defined Stage: A formal data governance framework is established, with clear policies, procedures, and standards. Roles and responsibilities related to data governance, including those of data stewards, are clearly defined and communicated across the organization. There’s an emphasis on training and awareness to ensure that the importance of data governance is understood at all levels.
- Managed Stage: Data governance policies and procedures are not just established but also actively enforced and monitored. Data governance becomes integrated with other business processes, ensuring that data management aligns with the organization’s overall goals. Data stewards play a proactive role, and there is a focus on measuring the effectiveness of governance initiatives.
- Optimized Stage: The organization reaches a level of maturity where data governance is an integral part of its culture. Advanced techniques and tools are used for proactive data quality and compliance management. Continuous improvement processes are in place, with data stewardship being a key component of strategic business decisions. The organization adapts quickly to new data regulations and technologies, and data governance drives innovation and business agility.
Metadata and Reference Data Management
Metadata and Reference Data Management is a critical aspect of comprehensive data management, focusing on the organization, classification, and standardization of data across systems to ensure accuracy, consistency, and context. Metadata, often described as "data about data," provides detailed information about the content, quality, condition, and other characteristics of data, facilitating its discovery, understanding, and effective use. Reference data, on the other hand, consists of standardized sets of values or codes used across an organization to categorize or classify information consistently. Together, these components play a pivotal role in enhancing data integrity, supporting data governance initiatives, and enabling seamless data integration and interoperability. By effectively managing metadata and reference data, organizations can improve data quality, streamline data-related processes, and unlock valuable insights, thereby driving better decision-making and strategic outcomes.
- Initial Stage: Organizations at this nascent phase possess a rudimentary understanding of metadata and reference data. Their management is often overlooked or handled in an ad-hoc manner, leading to inconsistencies and challenges in data utilization.
- Developing Stage: Recognition of the importance of structured metadata and reference data management begins. Efforts to document and standardize basic metadata and reference datasets are initiated, laying the groundwork for improved data governance and usage.
- Defined Stage: Formal policies and procedures for managing metadata and reference data are established, with clear roles and responsibilities defined. This structured approach ensures consistency in data interpretation and supports effective data integration strategies.
- Managed Stage: Metadata and reference data management practices are actively monitored and integrated into daily data operations. This stage sees the alignment of metadata and reference data with business processes, enhancing data accuracy and usability across the organization.
- Optimized Stage: At the pinnacle of maturity, organizations leverage advanced tools and methodologies for metadata and reference data management. Continuous improvement processes are in place, with metadata and reference data serving as key enablers for data-driven decision-making and strategic business initiatives.
Data Quality Management
Data Quality Management is a critical process within organizations aimed at ensuring the accuracy, completeness, consistency, reliability, and relevance of data throughout its lifecycle. This involves implementing controls, policies, and procedures to identify, understand, and correct flaws in data, as well as to prevent future data quality issues. By systematically monitoring, maintaining, and enhancing the quality of data, organizations can make more informed decisions, improve operational efficiency, and reduce risks associated with poor data quality, such as financial losses or diminished customer satisfaction. Effective Data Quality Management supports a range of business processes, from analytics and reporting to customer relationship management, by ensuring that stakeholders have access to trustworthy data. This, in turn, strengthens the organization's data-driven decision-making capabilities and contributes to achieving strategic goals.
- Initial Stage: In this stage, the organization faces inconsistent and unreliable data quality, often without realizing the extent of the issue. There is no formal process in place to manage data quality, leading to challenges in decision-making and operational inefficiencies. Data errors are common, and there is little understanding of how poor data quality impacts business outcomes.
- Developing Stage: The organization starts to recognize the importance of data quality. Initial efforts are made to identify and address data quality issues, but these efforts are often reactive and localized to specific departments or datasets. Basic monitoring systems might be introduced, but there is no organization-wide strategy for data quality management.
- Defined Stage: At this stage, the organization develops and implements standardized data quality measures. Regular data audits and cleansing processes are introduced to maintain and improve data quality. There is a greater understanding of the impact of data quality on business processes, and efforts are made to ensure that data is accurate, complete, and consistent across the organization.
- Managed Stage: Data quality management is now integrated into daily operations. The organization uses data quality metrics to tie data quality directly to business outcomes. Data quality initiatives are aligned with business objectives, and there is a focus on maintaining high-quality data as a part of the organizational culture. Employees at all levels understand their role in maintaining data quality.
- Optimized Stage: In the final stage, the organization employs predictive models and advanced techniques to manage data quality. There is a continuous refinement of data based on evolving business needs. The organization proactively addresses data quality issues before they impact business processes, and there is a strong emphasis on continuous improvement and adaptation to changing data environments.
Data Integration and Interoperability
Data Integration and Interoperability refer to the processes and technologies that enable the seamless sharing and consolidation of data across different systems, platforms, and environments within an organization. This dimension focuses on breaking down data silos to create a unified, coherent view of information, ensuring that data from disparate sources can be accessed, combined, and utilized effectively. Data integration involves techniques such as ETL (Extract, Transform, Load), APIs, and middleware solutions to aggregate and harmonize data, while interoperability ensures that different systems and applications can communicate and exchange data efficiently, using common standards and protocols. Achieving high levels of data integration and interoperability is essential for organizations looking to leverage comprehensive analytics, improve operational efficiencies, and deliver personalized customer experiences. It facilitates informed decision-making by providing a holistic view of data assets and supports agility by enabling flexible responses to changing business needs.
- Initial Stage: At this foundational stage, data is typically isolated within different departments or systems, leading to 'data silos'. Manual data integration processes are common, often resulting in significant inefficiencies, errors, and a lack of timely data access. The organization struggles with gaining a unified view of its data, which hampers decision-making and strategic initiatives.
- Developing Stage: The organization begins to recognize the drawbacks of data silos and starts initial efforts to integrate data sources. Basic ETL (Extract, Transform, Load) processes are introduced to consolidate data, but these processes may not be optimized and can be somewhat rudimentary. The challenge at this stage is to establish a foundational level of integration that can be built upon in later stages.
- Defined Stage: A more systematic and strategic approach to data integration is adopted. The organization may implement integration platforms to streamline the process, moving beyond basic ETL to more sophisticated techniques. This stage focuses on developing a robust integration framework that can handle diverse data sources and formats, aiming for consistency and accessibility of data across the organization.
- Managed Stage: Data integration is now a seamless part of the organization's operations. Advanced ETL processes, real-time data integration, and API-based integrations are utilized to ensure that data flows smoothly between systems and departments. The organization prioritizes interoperability, ensuring that different systems can communicate effectively and that data is readily available for various applications.
- Optimized Stage: The organization achieves a state of full interoperability and agility in its data environment. Integration processes are highly optimized, supporting advanced analytics capabilities and enabling real-time insights and decision-making. The focus is on maintaining a flexible and adaptive integration architecture that can quickly respond to new data sources, emerging technologies, and evolving business needs.
Data Storage and Architecture
Data Storage and Architecture encompasses the strategies, technologies, and frameworks used to store, manage, and organize data assets within an organization. This critical dimension of data management ensures that data is stored in a manner that is secure, scalable, accessible, and efficient, supporting both current operational needs and future growth. It involves selecting the appropriate storage solutions—such as databases, data warehouses, data lakes, and cloud storage options—based on the type, volume, and usage patterns of the data. The architecture aspect focuses on designing the data environment to optimize data flow, processing, and integration across various platforms and systems. Effective Data Storage and Architecture are foundational for enabling advanced analytics, ensuring high performance of data-driven applications, and maintaining data governance standards. By carefully planning and implementing robust data storage and architectural practices, organizations can ensure their data is protected, compliant with regulations, and readily available for insight generation and decision-making processes.
- Initial Stage: In this stage, organizations often struggle with disparate and inefficient data storage solutions. Data is stored in multiple locations and formats, leading to challenges in data accessibility, management, and security. There is a lack of cohesive strategy for data storage, which can result in increased costs, data loss risks, and difficulties in data retrieval and analysis.
- Developing Stage: Recognizing the limitations of their existing storage solutions, organizations start to consolidate data storage. This stage typically involves the introduction of more structured databases and initial efforts to centralize data storage. The focus is on improving data accessibility and reducing the inefficiencies of fragmented storage systems. However, scalability and long-term storage strategies may still be underdeveloped at this point.
- Defined Stage: The organization moves towards implementing scalable and more sophisticated storage solutions, such as data warehouses or cloud storage options. The aim is to accommodate growing data volumes and diverse data types while ensuring data security and compliance. This stage often involves a more strategic approach to data architecture, considering aspects like data lifecycle management, scalability, and integration with other systems.
- Managed Stage: Advanced storage solutions are fully implemented, featuring high availability, robust disaster recovery capabilities, and effective data backup processes. The focus shifts to optimizing these storage solutions for performance, cost-efficiency, and security. Data storage and architecture are closely aligned with the organization's broader IT and business strategies, ensuring that data supports and enhances business operations.
- Optimized Stage: At this stage, the organization achieves a highly efficient, cloud-based, and flexible data architecture. Data storage is not only about maintaining data but also about enabling dynamic access, analysis, and sharing. The architecture supports efficient data lifecycle management, adapting quickly to changing business needs and technological advancements. Emphasis is placed on leveraging cloud technologies for scalability, resilience, and innovation.
Data Security and Compliance
Data Security and Compliance are paramount in safeguarding an organization's data assets against unauthorized access, breaches, and other security threats, while also ensuring adherence to legal, regulatory, and ethical standards. This dimension involves implementing robust security measures such as encryption, access control, and network security protocols to protect sensitive and critical data. Additionally, it encompasses the development and enforcement of policies and practices that comply with data protection laws and industry regulations, such as GDPR, HIPAA, or CCPA. Ensuring data security and compliance is crucial not only for protecting the organization's reputation and financial health but also for maintaining trust with customers, partners, and regulators. It requires a continuous effort to monitor, assess, and update security practices and compliance measures in response to evolving threats and changing regulatory landscapes. Effective data security and compliance management help organizations navigate the complexities of the digital world, minimizing risks and creating a secure environment for data utilization and innovation.
- Initial Stage: Organizations in this stage have minimal data security measures in place, leading to potential risks of data breaches and non-compliance with regulatory standards. Data security is often an afterthought, and there's a lack of awareness about the importance of comprehensive data protection and compliance requirements. This stage is characterized by reactive responses to security incidents and compliance issues.
- Developing Stage: Recognizing the risks associated with poor data security, organizations start implementing basic security protocols and controls. There's a growing awareness of compliance requirements, but efforts to meet these standards are still in the early phases. The challenge here is to transition from a reactive to a more proactive security mindset, beginning to understand the implications of data breaches and non-compliance.
- Defined Stage: Formal data security policies and compliance frameworks are established. The organization is actively working to comply with legal and regulatory standards. Data security measures are more structured, and there's a concerted effort to educate employees about security best practices. Regular security assessments and compliance audits are introduced to ensure ongoing adherence to policies and regulations.
- Managed Stage: At this stage, data security and compliance measures are integrated into all aspects of data handling and business processes. The organization conducts regular audits and actively monitors for compliance with standards. There's a focus on continuously improving security measures and staying updated with the latest regulations and best practices. Security and compliance become ingrained in the organizational culture.
- Optimized Stage: The organization reaches a level where data security and compliance strategies are advanced and proactive. This includes continuous monitoring of the regulatory landscape and implementing cutting-edge security technologies and practices. There's an emphasis on anticipating and mitigating security threats before they can impact the organization. Compliance monitoring is continuous, and the organization swiftly adapts to new regulations.
Data Culture and Literacy
Data Culture and Literacy within an organization refer to the collective understanding, values, and practices that encourage the effective use of data in decision-making processes at all levels. A strong data culture promotes an environment where data is readily accessible and valued as a key asset, and where employees are empowered with the data literacy skills necessary to interpret, analyze, and utilize data correctly and ethically. Data literacy encompasses the ability to read, understand, create, and communicate data as information. Cultivating a data-driven culture and enhancing data literacy are essential for organizations aiming to leverage data for strategic insights, operational improvements, and competitive advantage. It involves not just training and education but also a shift in mindset and attitudes towards data, encouraging curiosity, critical thinking, and a willingness to experiment and learn from data. Building a robust data culture and literacy ensures that data-driven decision-making becomes ingrained in the organizational fabric, leading to more informed, efficient, and effective outcomes.
- Initial Stage: At this foundational stage, there is low awareness of the value of data within the organization. Data-driven decision-making is limited, often due to a lack of understanding about how to effectively use data. The organizational culture may not yet recognize the strategic importance of data, and there are minimal efforts, if any, to promote data literacy.
- Developing Stage: As the organization begins to recognize the importance of data, initial steps are taken to increase awareness and understanding of data's role in decision-making. This may include introductory training sessions on data handling and interpretation. The challenge at this stage is to start shifting the organizational mindset to appreciate the value of data-driven insights.
- Defined Stage: A more systematic approach to building a data-driven culture is adopted. Data literacy programs are rolled out organization-wide, ensuring that employees across different departments understand the importance of data and are equipped with the skills to interpret and use data effectively. The organization begins to integrate data usage into its standard operating procedures.
- Managed Stage: By this stage, a strong data-driven culture is established. Data literacy is deeply integrated into the organization at all levels, with ongoing training and development programs. Employees are encouraged to use data regularly in their work, and data-driven decision-making becomes a norm. Leaders play a key role in reinforcing a culture where data is valued and effectively utilized.
- Optimized Stage: In the final stage, the organization exhibits a continuous learning culture where data literacy is an integral part of strategic planning and innovation. Data is leveraged not just for operational decision-making but also for driving innovation and exploring new business opportunities. The organization continually adapts its data literacy programs to stay abreast of evolving data technologies and methodologies.
Analytics and Business Intelligence
Analytics and Business Intelligence (BI) encompass the technologies, tools, practices, and methodologies used to collect, integrate, analyze, and present an organization's raw data to create insightful and actionable business information. These processes empower decision-makers by providing a deep understanding of the internal and external environments in which they operate, enabling data-driven decision-making. Analytics ranges from descriptive (what has happened), through predictive (what could happen), to prescriptive (what should be done), leveraging statistical analysis, data mining, predictive modeling, and machine learning techniques. Business Intelligence, on the other hand, focuses on leveraging software and services to transform data into actionable intelligence that informs an organization's strategic and tactical business decisions. BI tools access and analyze data sets and present analytical findings in reports, summaries, dashboards, graphs, charts, and maps to provide users with detailed intelligence about the state of the business. Together, analytics and BI not only enhance operational efficiency and productivity but also provide a competitive edge by uncovering hidden opportunities, optimizing resource allocation, and improving customer experiences.
- Initial Stage: Organizations at this stage are at the beginning of their analytics journey. The use of data for decision-making is limited, often confined to basic, descriptive analytics. There is a lack of advanced tools and expertise, and analytics are not systematically integrated into business processes. The focus is mainly on historical data analysis, with minimal forward-looking insights.
- Developing Stage: The organization starts to recognize the value of analytics and begins to incorporate more regular use of analytics tools. This stage sees the introduction of business intelligence tools, though their use may be somewhat fragmented across different departments. The challenge here is to expand the use of analytics beyond basic reporting, beginning to explore more sophisticated analytical techniques.
- Defined Stage: At this stage, the systematic use of analytics in decision-making becomes more pronounced. The organization adopts a wider range of BI tools and techniques, and there is a concerted effort to train staff in analytics. Data starts to play a more integral role in strategic planning, and there is a focus on integrating analytics across various business functions.
- Managed Stage: Advanced analytics capabilities, including predictive and prescriptive analytics, are now in place. There is a strong alignment between analytics and business strategies, with analytics playing a key role in driving business decisions. The organization has the capability to not only understand past performance but also predict future trends and prescribe actions. The use of data and analytics is widespread and deeply embedded in the organizational culture.
- Optimized Stage: In the optimized stage, the organization is at the forefront of analytics capabilities. Cutting-edge techniques, including AI and machine learning, are fully integrated into the analytics processes. Data-driven innovation is a key focus, with analytics driving new business models and strategies. The organization leverages analytics for competitive advantage, continually exploring new ways to utilize data for strategic gain.
Conclusion
In conclusion, the journey through the Data Maturity Model is a continuous and evolving process that plays a crucial role in an organization's ability to harness the true power of its data assets. As we have explored, each stage of the model – from the Initial to the Optimized – presents its own set of challenges and milestones. Organizations must navigate these stages thoughtfully, implementing structured policies, enhancing data quality, integrating systems, and fostering a culture of data literacy and security.
Key to this journey is the recognition that progress in data maturity is not just a technical endeavor but a strategic one, deeply intertwined with the broader business objectives and goals. By adhering to the benchmarks laid out in each stage and continuously striving for improvement, organizations can unlock valuable insights, drive innovation, and maintain a competitive edge in an increasingly data-centric world.
As you reflect on your organization’s position within this model, remember that the path to data maturity is unique for each organization. It requires a tailored approach that considers your specific business context, challenges, and goals. The journey might be complex and demanding, but the rewards – in terms of improved efficiency, decision-making, and strategic insight – are substantial.
Embrace the journey, invest in the right resources, and commit to continuous learning and adaptation. With these practices, your organization can not only achieve data maturity but also leverage its full potential to propel your business towards new heights of success.
Member discussion