The Cost of Poor Data Quality: Understanding the Impact on Business
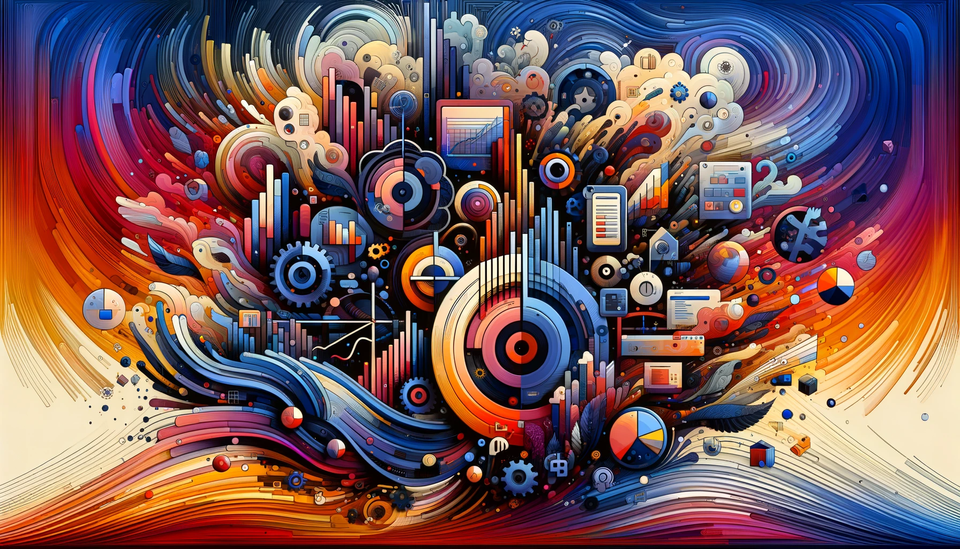
Introduction
In the digital age, data stands as the cornerstone of business decision-making, driving strategies, innovations, and customer engagements across industries. As businesses increasingly rely on data to navigate competitive landscapes, the quality of this data becomes paramount. Yet, in the vast ocean of information that modern enterprises swim in, poor data quality emerges as a significant and often underestimated challenge.
This article sheds light on the often-overlooked issue of poor data quality and its far-reaching implications for businesses. While data holds the power to unlock new opportunities and drive growth, its value is deeply intertwined with its quality. Data errors, inaccuracies, or outdated information can lead to misguided decisions, financial losses, and tarnished reputations.
As we navigate through the intricacies of data quality, we will explore what it means for data to be of high quality, the common pitfalls businesses face in maintaining this quality, and the multifaceted impact of subpar data on operational efficiency, financial health, legal standing, and customer relations. This journey is not just about recognizing the pitfalls of poor data quality but also about understanding the essential role of robust data management in building a resilient and thriving business.
In an era where data is a critical asset, understanding and mitigating the risks associated with poor data quality is not merely an operational necessity—it's a strategic imperative.
Understanding Data Quality
Data quality is a multifaceted concept, crucial for the effective functioning of any business. At its core, data quality refers to the suitability of data to serve its intended purpose. High-quality data should be accurate, complete, relevant, and timely, enabling businesses to make informed decisions.
Accuracy
Accuracy is the cornerstone of data quality. It refers to the correctness of data in representing the real-world entities or events it is supposed to depict. Accurate data must be free from errors, whether they stem from incorrect data entry, outdated information, or misinterpretation during data collection.
Completeness
Data completeness means that no critical pieces of information are missing from the dataset. Incomplete data can lead to misleading conclusions and flawed decision-making.
Relevance
Relevance ensures that the data collected and maintained is pertinent to the specific needs of the business. Data that is not relevant can clutter systems, making it harder to extract valuable insights.
Timeliness
Timely data is up-to-date and available when needed. Decisions based on outdated information can be as detrimental as those based on inaccurate data.
Understanding these dimensions of data quality is vital for businesses. High-quality data can lead to better strategic decisions, improved operational efficiency, and enhanced customer satisfaction. Conversely, poor data quality can have the opposite effect, undermining the very foundations on which business decisions are made. This section sets the stage for exploring the common data quality issues businesses face and their impact on various aspects of organizational performance.
Common Data Quality Issues
Navigating the complex landscape of data management, businesses frequently encounter a range of data quality issues. These problems can undermine the integrity of data and significantly impact business operations. Understanding these common issues is the first step towards mitigating their effects.
Data Duplication
One of the most prevalent issues is data duplication. This occurs when identical pieces of information are stored in multiple places, leading to confusion and inefficiency. For instance, a customer might be listed several times in a database with slightly different details, causing challenges in customer relationship management and marketing efforts.
Inaccurate Data
Inaccuracies in data can stem from various sources, such as human error during data entry, outdated information, or incorrect data importation. This can lead to faulty analysis and misguided business decisions. For example, inaccurate sales data can mislead a business about its market performance, impacting strategic decisions.
Data Decay
Data decay refers to the gradual degradation of data quality over time. As the world changes rapidly, information that was once accurate becomes outdated. This is particularly prevalent in dynamic fields like contact information, where changes are frequent and inevitable.
Inconsistent Data Formats
Inconsistency in data formats across different systems can pose significant challenges, especially in large organizations that use multiple data management systems. Such inconsistencies complicate data integration and analysis, leading to inefficiencies and potential errors.
Poorly Defined Data
Ambiguity in data definitions and lack of standardization can lead to misinterpretation and misuse of data. Clear and consistent definitions are crucial for effective data utilization.
Lack of Contextual Data
Data without sufficient context or background information can be misleading. Contextual data is key to understanding the nuances and implications of the data, ensuring accurate interpretation and decision-making.
These common data quality issues, if not addressed, can have a cascading effect on various aspects of business operations, strategy, and decision-making. Recognizing and rectifying these issues is crucial for maintaining the integrity and usefulness of business data. In the following sections, we will delve into how these issues impact business operations, financial health, and customer relationships.
Impacts on Business
The ramifications of poor data quality are extensive, permeating various facets of a business. From operational efficiency to strategic decision-making, the impact of subpar data can be both profound and far-reaching.
Operational Efficiency
Poor data quality directly affects the day-to-day operations of a business. When data is inaccurate or incomplete, operational processes can become inefficient and error-prone. For example, in a supply chain context, inaccurate inventory data can lead to overstocking or stockouts, disrupting the entire supply chain and affecting customer satisfaction.
Financial Health
The financial implications of poor data quality are significant. On a direct level, businesses may incur substantial costs in correcting data errors, reconciling discrepancies, and dealing with the consequences of decisions made based on inaccurate data. Indirect costs include lost revenue opportunities due to misinformed business strategies or ineffective marketing campaigns driven by flawed customer data.
Decision-Making
Strategic decision-making relies heavily on data. When the quality of data is compromised, so are the decisions based on it. Executives may make strategic errors, such as misjudging market trends or customer needs, if their decisions are rooted in inaccurate data. This can lead to misguided investments, product development missteps, and missed market opportunities, impacting the company's long-term strategic positioning.
Competitive Disadvantage
In the fast-paced business world, the ability to make quick, informed decisions is a competitive advantage. Poor data quality slows down this process, hindering a company's ability to respond swiftly to market changes and competitor moves. In contrast, businesses with high-quality data can leverage it for predictive analytics and trend analysis, gaining a competitive edge.
Compliance and Risk Management
Poor data quality also poses risks in regulatory compliance and risk management. In industries where regulatory compliance is critical, inaccurate data can lead to violations and legal repercussions.
The impact of poor data quality on a business is multifaceted, affecting its operational efficiency, financial health, strategic decision-making, competitive stance, and compliance posture. Addressing data quality issues is not just about correcting errors; it's about building a foundation for sustainable business growth and resilience. In the next section, we will explore the legal and compliance risks associated with poor data quality, further underscoring its significance in the business world.
Legal and Compliance Risks
The realm of data quality extends beyond operational efficiency and financial performance, encompassing legal and compliance risks that can have profound consequences for businesses.
Regulatory Compliance
In many industries, particularly finance, healthcare, and telecommunications, regulatory bodies enforce strict standards for data management. Compliance with these regulations requires data to be accurate, complete, and verifiable. Failure to comply due to poor data quality can result in legal actions, hefty fines, and damage to reputation. For instance, financial institutions must adhere to Anti-Money Laundering (AML) and Know Your Customer (KYC) regulations, which demand high accuracy in customer data.
Data Privacy Laws
With the advent of data privacy laws like the General Data Protection Regulation (GDPR) in Europe and the California Consumer Privacy Act (CCPA) in the U.S., the way businesses handle personal data has come under intense scrutiny. These laws mandate the accurate handling, processing, and storage of personal data. Inaccuracies in data, especially personal data, can lead to breaches of these laws, attracting significant penalties and loss of customer trust.
Risk Management
Accurate data is crucial for effective risk management. Inaccurate data can lead to flawed risk assessments, resulting in inadequate preparations for potential issues. For businesses, this means increased vulnerability to market fluctuations, credit risks, operational failures, and other unforeseen challenges.
In summary, the legal and compliance landscape underscores the criticality of maintaining high-quality data. Navigating this complex terrain requires a diligent approach to data management, ensuring not only compliance with various regulations but also safeguarding the business against legal and reputational risks.
Effects on Customer Relations
The quality of data held by a business significantly influences its relationship with customers. In an era where customer experience is paramount, the accuracy, relevance, and timeliness of data can greatly enhance or detrimentally impact this dynamic.
Personalization and Customer Engagement
One of the key areas where data quality plays a pivotal role is in personalization and customer engagement strategies. Accurate and up-to-date customer data allows businesses to tailor their communication and services, leading to more effective marketing and improved customer satisfaction. For instance, accurate purchase history and preference data enable businesses to offer relevant product recommendations, enhancing the shopping experience.
Trust and Reliability
Customers entrust businesses with their personal information with the expectation of privacy, security, and beneficial use. Inaccurate data can lead to breaches of this trust, such as sending incorrect or irrelevant communication, which can be perceived as intrusive or negligent. Ensuring data accuracy helps maintain customer trust and demonstrates a business’s commitment to respecting and valuing its customers.
Customer Service Quality
In customer service, the quality of data directly impacts the quality of service delivered. Accurate customer records ensure that service agents have the right information to address customer issues effectively and efficiently. In contrast, poor data quality can lead to miscommunication, unresolved issues, and ultimately, customer dissatisfaction.
Building Long-term Relationships
The cumulative effect of interactions based on high-quality data can lead to stronger, longer-lasting customer relationships. Customers are more likely to remain loyal to a brand that consistently recognizes and respects their preferences and needs through accurate data usage.
In summary, the quality of customer data is a crucial determinant of the strength and depth of customer relationships. By prioritizing data quality, businesses can significantly enhance customer engagement, build trust, and foster loyalty. This, in turn, leads to better customer retention, higher customer lifetime value, and a stronger brand reputation.
Conclusion
As we have explored in this article, the impact of poor data quality in the business realm is far-reaching and multifaceted. From operational inefficiencies to strategic missteps, financial losses, legal risks, and weakened customer relationships, the repercussions of subpar data are profound. It becomes clear that data quality is not merely a technical issue but a strategic business imperative.
The importance of investing in robust data management practices cannot be overstated. Businesses must recognize that the journey to high data quality is continuous and requires a commitment to meticulous data governance, regular audits, and a culture that values accuracy and reliability. The strategies to improve data quality—ranging from employing advanced data management tools to training personnel and aligning organizational processes with data quality objectives—are essential investments in the long-term health and success of a business.
In conclusion, high-quality data is the lifeblood of modern businesses. It empowers informed decision-making, drives operational efficiency, ensures compliance, and strengthens customer connections. As businesses continue to navigate a data-driven world, prioritizing data quality will be key to maintaining a competitive edge, achieving sustainable growth, and building enduring customer relationships. The cost of poor data quality is too high for any business to overlook.
Member discussion